The ART© of Transformation
(Accelerated, Risk-based Transformation)
ART© is designed to facilitate and accelerate operational transformation as an iterative process with a risk-based methodology and by adopting established concepts such as operational risk assessment, underwriting appetite or investment management strategies. ART© has been initially designed as a response to the digital transformation gap in the (re)insurance sector, also amplified by difficulties to integrate new options from insurtechs in insurance core processes. ART© can easily be adapted to any other area and/or industry.
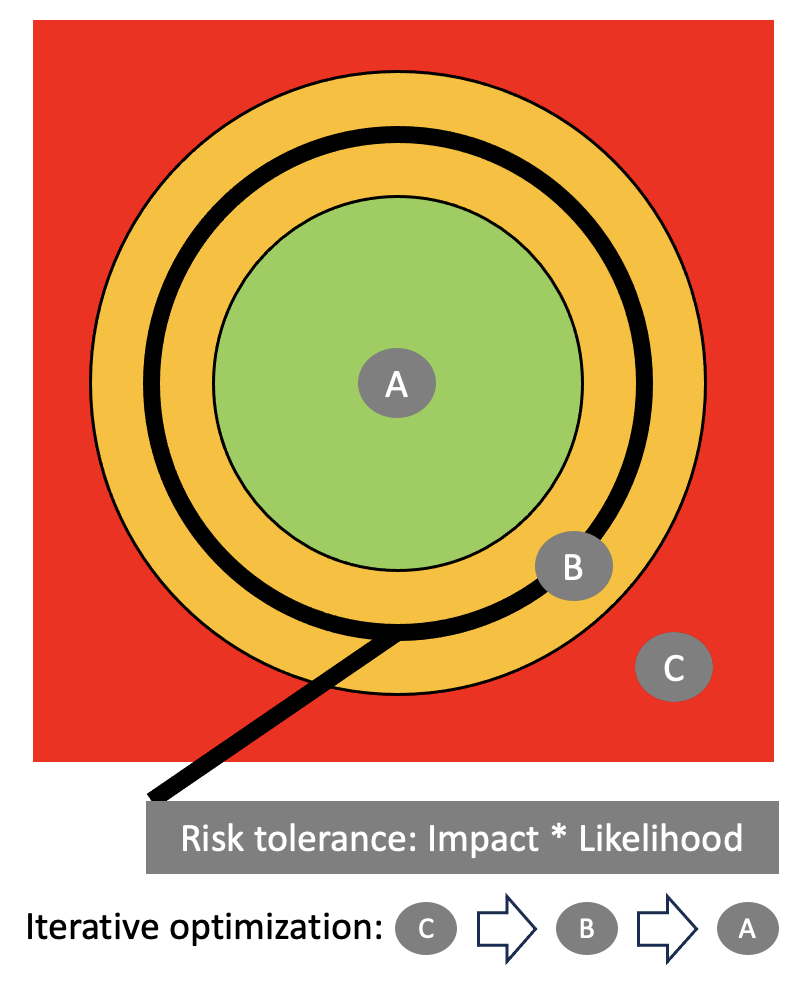
How to take and actively manage transformation risk?
Goals of iterations:
Optimize risk tolerance
Increase number of transactions in cluster A
Reduce issues and efforts for cluster B
Develop solution for remaining cluster C (post-transformation)
Sensitize ecosystem to ongoing transformation
Move from culture of failure to risk culture
"Learn to walk before you run"
Iterative steps (standard):
Agree on risk tolerance with management
Define parameters for risk clusters A, B and C
Prepare reactions for detected issues in cluster B
Apply target solution over defined period
Track & manage cluster B; spot-check cluster A & C
Quantitative and qualitative review for all clusters
Optimize target solution
Copyright © 2024 by Oaswiss AG
Example: Automated e-mail response by using CompanyGPT
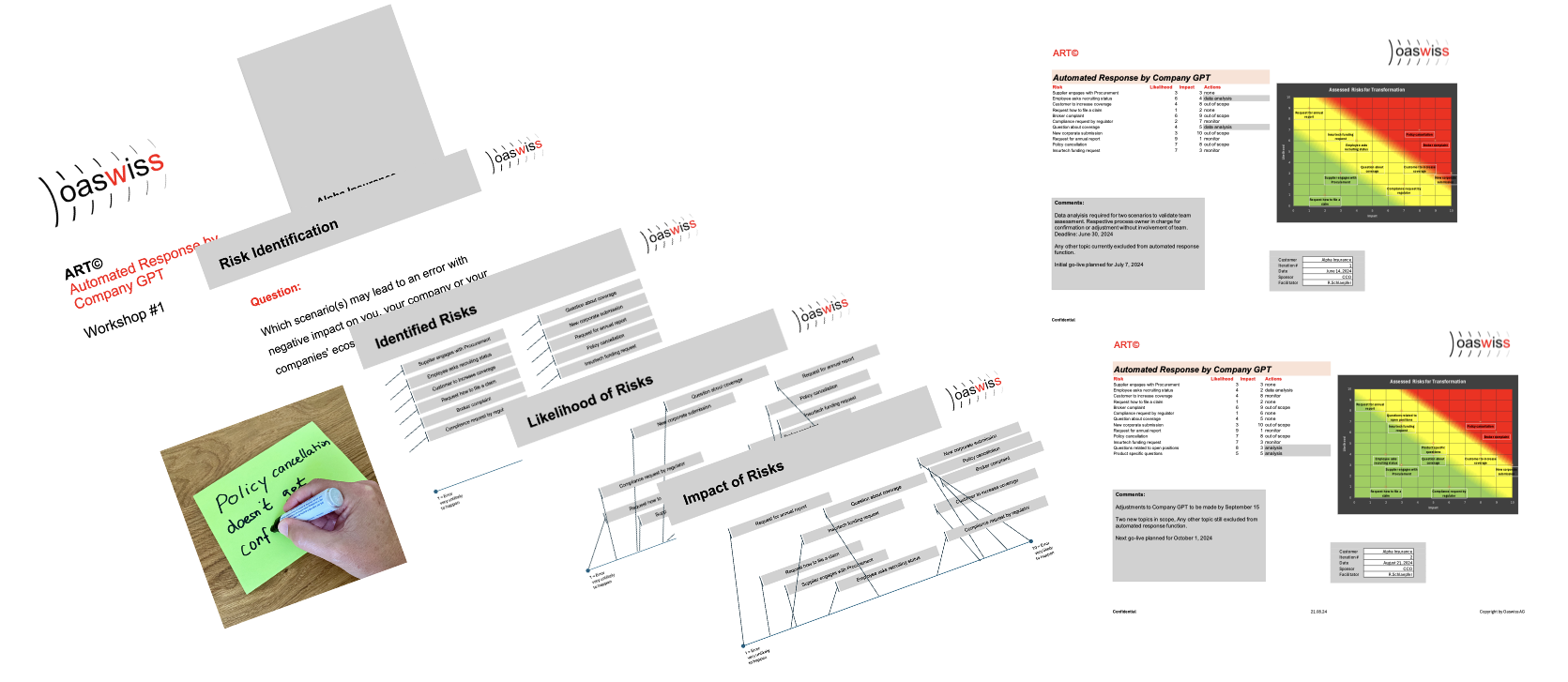